Blogs / o4-mini: OpenAI’s Small and Powerful Reasoning Model for Lightweight Applications
o4-mini: OpenAI’s Small and Powerful Reasoning Model for Lightweight Applications
May 3, 2025
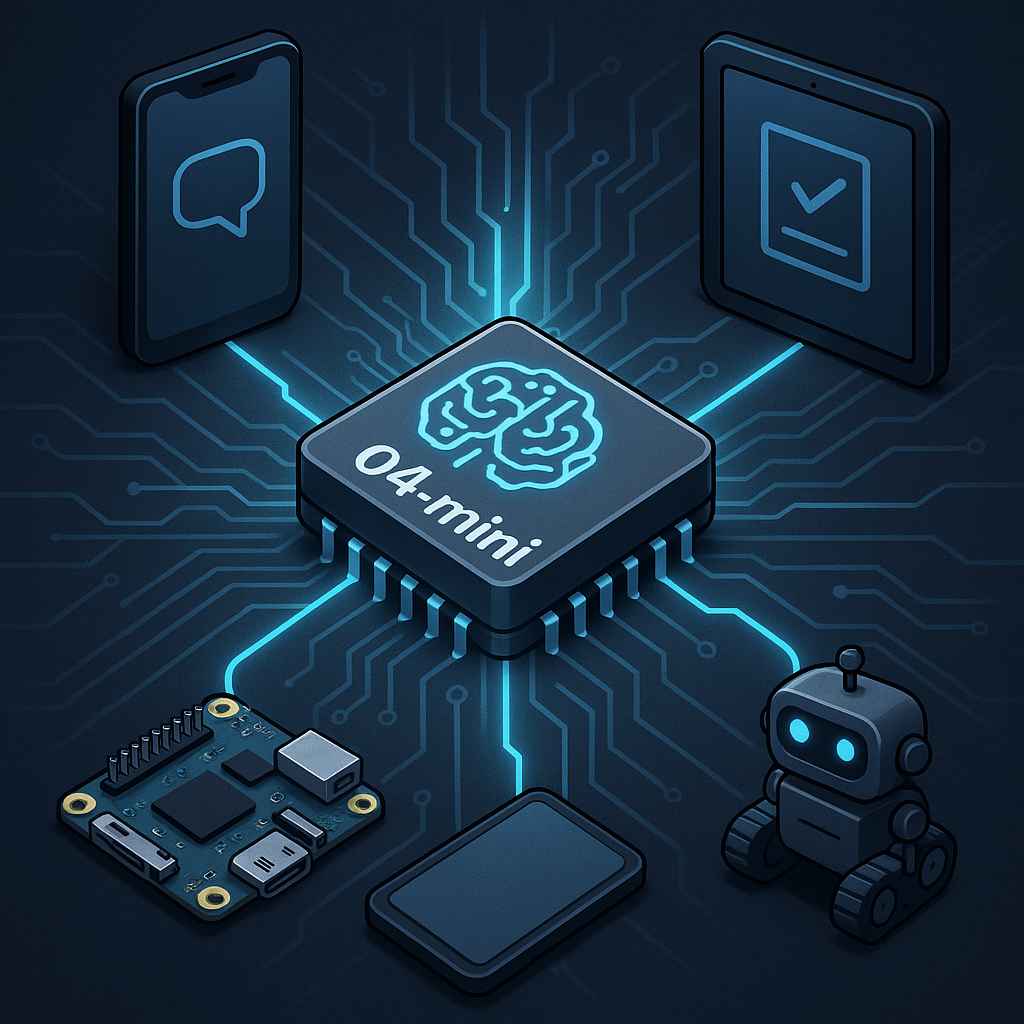
Introduction
In the rapidly growing world of artificial intelligence, optimizing models to deliver high performance with minimal resource consumption is one of the main challenges for developers. OpenAI introduced o4-mini, aiming to provide a compact and lightweight model for reasoning and response in streamlined applications. o4-mini is a smaller variant of the o4 reasoning series that maintains adequate accuracy and speed while using fewer resources. This article offers a comprehensive review of o4-mini, its features, advantages, disadvantages, applications, and usage.
History and Introduction of o4-mini
Development of OpenAI Reasoning Models
From the introduction of the first GPT model to the o1 series and o3-mini, OpenAI has continually enhanced its reasoning and analytical capabilities. The o4 models are known for their computational power and ability to solve complex problems. However, the large versions of these models require massive GPU resources and incur high operational costs.
Motivation Behind o4-mini
For many businesses and developers, using large models is neither economically nor technically feasible. By focusing on lightness and optimization, o4-mini enables leveraging the reasoning power of o4 with reduced cost and resource usage.
Architecture and Design of o4-mini
Transformer Structure
Like other o4 series models, o4-mini is based on the Transformer architecture. However, the number of layers and parameters in o4-mini has been reduced to minimize model size and memory usage.
Lightweight Optimizations
OpenAI employed techniques such as pruning, weight compression (quantization), and knowledge distillation to remove a substantial portion of the model size without accuracy loss. The result is a model that consumes less than 10% of the standard o4’s volume.
Model Parameters and Dimensions
-
Number of Layers: 24 layers compared to 60 in the base o4
-
Parameters: approximately 1.5 billion parameters (versus 175 billion in the large o4)
-
Memory Requirement: under 4 GB VRAM for inference
Performance and Accuracy of o4-mini
Comparison with Base o4
In light to medium reasoning tests, o4-mini retains approximately 90–95% of the accuracy of the original o4. For simple tasks or small datasets, the difference is negligible. However, in ultra-advanced benchmarks, a slight drop in performance may be observed.
Inference Speed
Due to its smaller size, o4-mini offers up to 3× faster inference than the base o4. This boost is crucial for real-time and mobile applications.
Resource Consumption
-
CPU-Only: capable of running on standard hardware
-
Small GPU: a 4–6 GB VRAM GPU is sufficient
-
Edge/IoT Usage: its low footprint allows embedding in edge devices
Applications of o4-mini
Lightweight Chatbots
Ideal for websites or apps requiring simple interactive responses, o4-mini can handle general questions, FAQs, and light conversations with good quality.
Basic NLP Tasks
Tasks such as text classification, keyword extraction, lightweight sentiment analysis, and short summarization are performed quickly and efficiently by o4-mini.
Office Automation
In office automation systems like email responses, support ticket management, or short report generation, o4-mini can easily run on low-power servers.
Mobile and Desktop Apps
Developers can integrate o4-mini as the AI engine in mobile or desktop apps with minimal memory and CPU requirements.
Lightweight Research and Analysis Tools
For quick text analysis, instant summarization, or rapid information extraction from short documents, o4-mini is an excellent choice.
Advantages and Disadvantages of o4-mini
Advantages
- Minimal size and resource consumption
- High inference speed
- Accuracy close to large models in lightweight use cases
- Runs on standard hardware and edge devices
- Lower infrastructure and power costs
Disadvantages
- Lower accuracy in heavy scientific and reasoning tasks
- Lacks support for some complex chain-of-thought functions
- Limited in generating long, highly structured texts
Security and Safety Considerations
OpenAI has also implemented reflective alignment and content filters in o4-mini to reduce the risk of generating harmful or inappropriate outputs. However, in sensitive domains like medical or legal fields, model outputs should always be human-reviewed.
Future of o4-mini and Upcoming Developments
Accuracy Improvements in Future Releases
OpenAI plans to enhance medium-level task accuracy to 98% of the large o4 model using new distillation techniques and enriched training metadata.
Release of Domain-Specific Versions
Domain-specific o4-mini versions for healthcare, legal, and finance applications are expected to be released independently.
Integration with Low-Code Tools
Ready-to-use packages and components for low-code/no-code platforms like Bubble and Retool are on the way, enabling non-technical developers to leverage o4-mini.
Conclusion
o4-mini represents a significant step toward lightweight reasoning models. With minimal resource consumption, high speed, and strong accuracy in lightweight applications, this model is an ideal solution for businesses and developers seeking a cost-effective and efficient AI tool. As specialized versions are released and continuous improvements are made, o4-mini has the potential to serve as the backbone of your lightweight AI—from mobile and edge devices to low-power servers.
✨ With DeepFa, AI is in your hands!! 🚀
Welcome to DeepFa, where innovation and AI come together to transform the world of creativity and productivity!
- 🔥 Advanced language models: Leverage powerful models like Dalle, Stable Diffusion, Gemini 2.5 Flash, Claude 3.7, GPT-o1, and more to create incredible content that captivates everyone.
- 🔥 Text-to-speech and vice versa: With our advanced technologies, easily convert your texts to speech or generate accurate and professional texts from speech.
- 🔥 Content creation and editing: Use our tools to create stunning texts, images, and videos, and craft content that stays memorable.
- 🔥 Data analysis and enterprise solutions: With our API platform, easily analyze complex data and implement key optimizations for your business.
✨ Enter a new world of possibilities with DeepFa! To explore our advanced services and tools, visit our website and take a step forward:
Explore Our ServicesDeepFa is with you to unleash your creativity to the fullest and elevate productivity to a new level using advanced AI tools. Now is the time to build the future together!